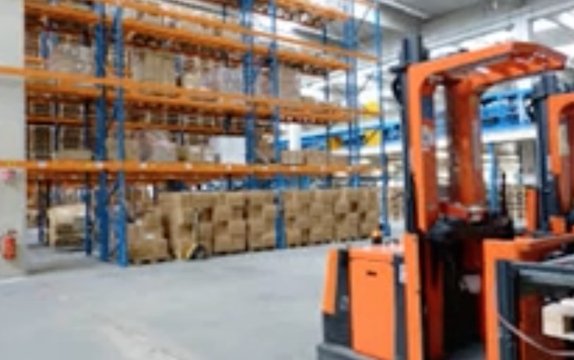
Dual Machine Learning
Solution Overview
Data Ingestion – Vendor operational data gathered from various Source Systems across geo locations was ingested into Azure storage
Data Modeling – Custom ML Data Models were built to forecast diverse gaps across several buckets
Data Insights – Predict the imminent red flags for different silos
Solution Benefits
- Effective planning of Inventory, Spare Parts, & other Supply metrics
- Effective maintenance of Vendor Performance & Vendor Credential metrics
- Predictive Demand Planning & Mapping
Our Approach – The solution used a custom dual ML algorithm to predict the overall diversions pertaining Vendors, Supply and Inventory. Generated proactive alerts for each buckets highlighting the possible event such as overuse of Tier Vendors, Inconsistent Payments, etc.
4 Phased Analysis – Data Science Project
- DATA ANALYSIS : Supply – Analyse and garner a Consolidated view of Purchasing . Determining Buyer Patterns such as – Who is buying & from who ? ( Both Regional and Global Level ). Analysis of Primary and Secondary Back Up Vendors & Vendor Data Consolidation. At the data level – Analysis and Consolidation of all supplies from individual suppliers. Analysis of avenues to look at reducing the no. of suppliers that are there in the portfolio.
- DATA ANALYSIS : Payments – Analysis of Payment Profiling per Vendor. Analysis of Payment Disbursements (Assumptions vs Reality). Analysis of Payment Scheduling . Analysis of Delivery Schedule mapped to Payment Disbursals. Analysis of Consistency in Payment Terms Adherence.
- DATA ANALYSIS : Vendor Performance – Data garnering, Analysis and Consolidation of Registered Vendors and Suppliers. Data Analysis of how individual vendors are being used. Correlation Analysis of Primary and Secondary vendors in terms of their use/overuse/underuse there might be an overuse of Primary/Secondary Vendors. Analysis of Vendor Teams for quick ramp ups or ran downs. Delivery Data Analysis to analyse delivery schedules, how close do vendors deliver as per schedule and generation of vendor performance. Analysis and Categorisation of Vendors in terms of reliability of delivery.
- DATA ANALYSIS : Demand Planning – Analysis of Vendor behaviours. Categorisation of Vendors based on delivery timeline adherence, etc.) Analysis of Vendors based on historical usage, to determine which vendor would be needed and when. To join those two together, to analyse and get to production inventory, to ensure supply of something when client needed . This part of the analysis attributes comes from Accountability side of the vendor (If the vendor is unreliable, Timeline Adherence, etc.). In accordance vendor flagging is mapped and integrated to stocks availability. Thereby determining, Who needs to supply and when and what’s the likelihood of it arriving on time.